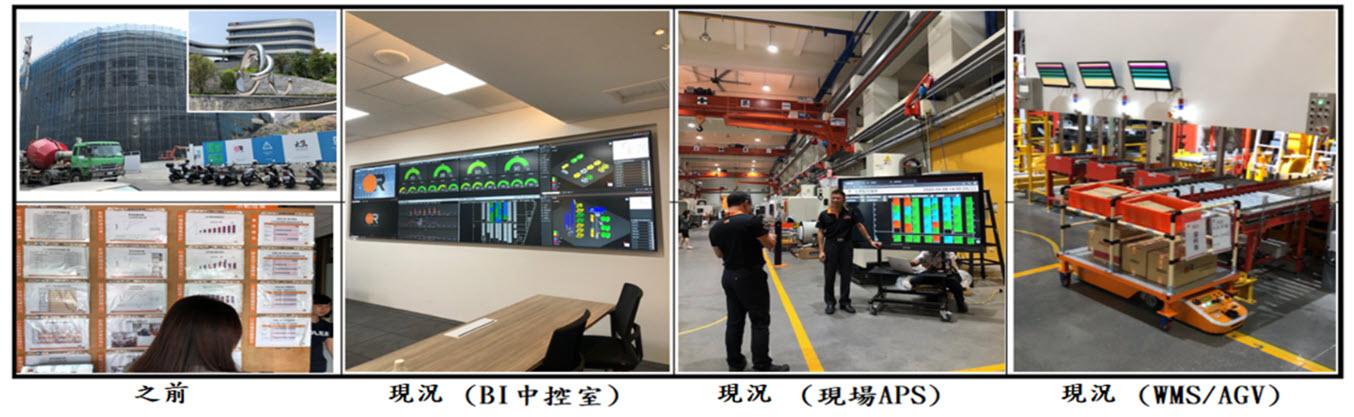
Problems
- Lack of communication between the original software supplier and in-house manufacturing engineers regarding practical application requirements.
- High costs associated with software updates.
- Delays of nearly a year before and after reform, leading to poor timeliness.
- Inaccurate personnel management. Manual production scheduling leads to inaccurate predictions.
- Lack of transparency and real-time access to production data.
- Inaccurate and untimely reporting, leading to inability to determine actual product costs.
- Inaccurate predictions of machine utilization rates and load rates. Poor control over Overall Equipment Effectiveness (OEE) and maintenance transparency.
- Delayed handling of production abnormalities, leading to idle labor and materials.
- Untimely feedback on work order losses and inaccurate data.
- Inaccurate material flow tracking, resulting in inability to know the location of materials and work-in-progress items in real time.
- Too much time spent collecting data during review meetings, leading to inability to make timely decisions.
- Excessive manual statistics collection leading to loss of working hours.
- Use of different heterogeneous systems resulting in many information silos and inability for systems to communicate with each other.
- Lack of system integration in the factory system, high reliance on personnel, and inability to support rapid expansion of company scale.
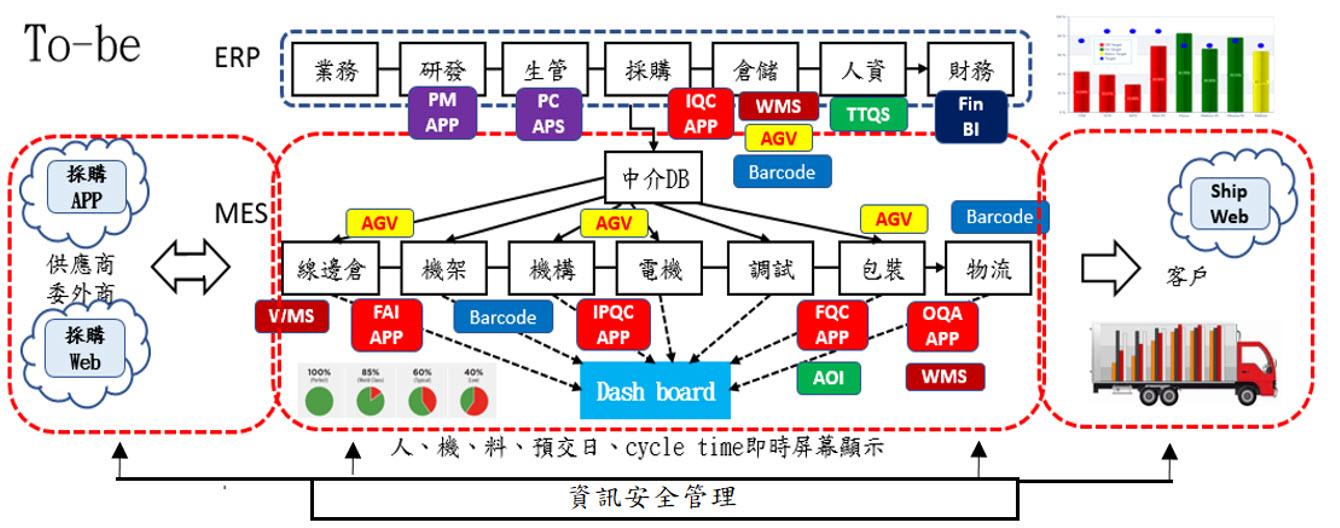
Solutions
Developed a machine networking integrated digital management system, with digital signals connected to OPTO 22 industrial computers operating on the OPC UA communication protocol. Completed equipment output CNC verification parameters according to different auxiliary machine specifications, displayed them in real-time via BI graphical dashboards, and indicated deviations or low standards with red lights and alarm displays, optimizing the quality of auxiliary machine management.
Under defined production capacity constraints, bound production orders to mathematical algorithm modules to perform mathematical calculations, interlocking, and transfers of personnel, machinery, materials, production cycles, and procurement operations. Input data via an app to instantly present material requirements (MRP) and capacity requirements on the war situation dashboard.
Developed Statistical Process Control (SPC) modules for online control through e-APP, establishing key quality monitoring processes such as First Article Inspection (FAI), Incoming Quality Control (IQC), In-Process Quality Control (IPQC), and Final Quality Control (FQC) based on customer QA standards.
In the Product Lifecycle Management (PLM) module, established a Bill of Tooling (BOT) to manage the usage frequency of tools, jigs, equipment, and molds through predetermined maintenance instead of regular maintenance, maintaining the accuracy and availability of tooling checks, ensuring PLM (available time) > Cycle Time.
Displayed stoppages, shutdowns, material shortages, quality defects, and procurement failures with red lights on the Gantt chart dashboard of Advanced Production and Scheduling (APS), allowing production management to quickly adjust production orders and internal resources, updating production schedules on the war situation dashboard in real-time, and displaying various BI charts for visual management.
Derived the Idle Index (KII) from the formula Total Equipment Efficiency (OEE) = Performance Index (P) * Availability Index (A) * Quality Index (Q), using KII as an indicator for profit and loss assessment, further adjusting internal resources in real-time and making quick decisions.
Target Audience
- Machinery, rubber injection molding, and automation equipment industries.
- Factories undergoing IT/OT/DT integration, aiming for smart factory upgrades.
Software Specifications
Establishment of a Kanban management system: Utilize “On-site Tablet Production Kanban,” “Production Progress Kanban,” and “Abnormality Kanban” to achieve a management mode driven by on-site Kanban, shifting from front-end producers determining production quantities to back-end customer demand. Stations can transmit messages through “Visual Kanban,” including tracking production progress, production time, inventory locations, on-site production performance, etc. Once abnormalities occur in production, relevant personnel can be notified immediately and take measures to resolve issues.
Implementation of Just-in-Time (JIT): JIT centers on “punctual production,” producing the right quantity of high-quality products at the appropriate time according to customer order requirements. Utilize an APS advanced scheduling system based on “pull” production to precisely control daily production capacity and demand. Equipment and personnel schedules can be automatically arranged, and production management personnel can adjust monthly and daily plans flexibly based on rush orders and on-site abnormal conditions, minimizing idle time on the production line and ensuring smooth and balanced semi-finished product flow between each workstation, known as “balancing” in JIT.
Implementation of Jidoka: Jidoka involves two aspects: “automation” of product manufacturing and “autonomy” of production quality control, i.e., “endowing machines with human intelligence.” If quality abnormalities occur in the production line, on-site personnel can stop machine operation to prevent defective products from entering the next process. Parameters for molding can be automatically recorded via networking of injection molding machines. The system will automatically send abnormal alerts when quality deviations occur. Inspection equipment can be networked into an SPC system for quality monitoring and early warning notifications.
Establishment of standardized operations: The machinery industry establishes a Product Data Management (PDM) system and a Product Lifecycle Management (PLM) system to digitize Standard Operating Procedures (SOP) for products. This can improve on-site operational efficiency, ensure product quality, reduce errors, and achieve cost reduction and continuous improvement goals.
Elimination of the seven wastes: Eliminating waste refers to any non-value-added activities, including overproduction, waiting time, transportation, inventory, motion, defects, and underutilization of employee potential. The machinery industry can reduce equipment downtime by automatically notifying equipment abnormalities through equipment networking or on-site reporting APP systems. Implementing WMS warehouse management systems for material batch and First-In, First-Out (FIFO) control to reduce inventory waste. Utilize SPC statistical process control systems to issue warnings for on-site quality abnormalities, reducing defective product waste.
Production equipment management: a. Acquisition of processing condition data b. Acquisition of equipment data (utilization rate) c. Production command system (work order allocation, optimization, simulation, capacity load assessment) d. Real-time war situation dashboard system WMS management of material and production logistics e. Tooling, fixture, mold, and cutting tool PLM management tracking